How Text Annotation Is Helping Companies Succeed by Monitoring Social Noise
Data is growing enormously, with 2.5 quintillion bytes generated by internet users daily. Social media users are expected to grow from 4 billion in 2022 to 6 billion by 2025. Due to social media inclination and empowerment, people love to share their opinions on various platforms.
Whether it's a rant about the Wi-Fi at a coffee shop or a heartfelt post about a life-changing event, people like to express themselves online. And this can be great news for companies who are looking to understand and respond to public sentiment.
Through text annotation, companies can monitor social media for discussion around their brand or product and then quickly and easily respond to any concerns or praise from customers. This is helping many businesses succeed by monitoring social noise - read on to find out how text annotation is supporting industries to translate social response and accelerate services.
What Is Data Annotation?
Modern AI and ML solutions depend upon labeled input data for training and learning models. Data annotation or labeling is the process of categorizing or labeling input data such as images, videos, audio, and text files.
Without data annotation, machines cannot completely understand information and identify attributes. Human data annotators have to play their role in making the identification and classification of useful data from the input easier for the machines.
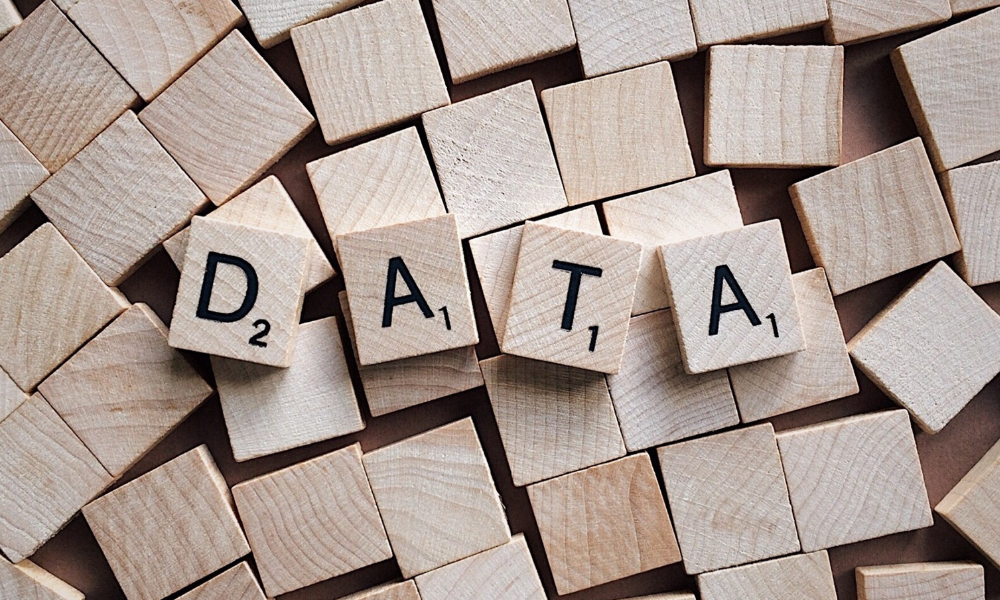
What Is Text Annotation?
Text annotation is the most common labeling technique among all data types, with most companies relying on text for ML procedures. Text annotation uses tags to highlight sentences, phrases, or keywords from the text for training machines to recognize human expressions and emotions.
The sentiments or expressions highlighted by the text annotation process are fed as training data for the machines to better process and handle natural human language and digital conversations. Text annotation builds a communication channel between humans and computer models. Incorrect annotations can lead to misinterpretation of the text and machines producing garbage outputs.
Why Is Text Annotation Important?
According to Statista, revenues from natural language processing will hit around $43 billion. Text annotation forms the foundation of natural language processing. Therefore, it should be explored and considered an essential pillar for growth. The covid-19 pandemic and the development of e-commerce marketing strategies have shifted consumer trends and increased demand for digital solutions.
Text annotation is an important practice for organizations interested in translating consumer demands and incorporating feedback into their services. Effective and efficient annotation can help produce accurate results, improving customer experience and enhancing satisfaction. Moreover, it enables organizations to reach their targets by understanding user needs and reducing the time and effort of human annotators to translate text and form solutions.
Text Annotation Types
Text annotation offers different mechanisms for tagging and labeling text. Text annotation techniques can be categorized into the following branches:
Named Entity Tagging (NET)
Named entity tagging or recognition is used for labeling certain words with proper names or predefined categories from the text. NET helps detect entity information, like name, time, location, etc., from large datasets that human annotators have annotated or labeled.
Data divided into categories is easier for machines to handle. Machines can better recognize the actual purpose behind a query, process it the right way, and get it resolved on time. Social media applications or platforms can use named entity tagging to tag different users, places, and businesses to assist e-commerce clients and promote their products.
Intent Annotation
With the development of AI and automation, the application of chatbots is gaining tremendous popularity. The human-machine conversation interfaces need to be capable of understanding plain language and user intent now more than ever. Intent annotation observes the intentions behind the text or conversation and categorizes it as a request and command to process it.
When the machine or bot fails to recognize the intent, it can no longer proceed with the request and would require the text to be restructured or rephrased. If the bot can still not comprehend the text after rephrasing, it would forward the query to the human agent, defying the entire purpose of automation and machine utilization. Chatbots deployed in customer service need to understand the purpose of conversation to provide effective services.
Semantic Annotation
Semantic annotation adds certain documents with relevant information to the content. It involves attaching metadata to a text document, such as people, companies, products, places, etc. Semantic annotation helps the customers find the right product for themselves by improving item listings.
Semantic annotation attaches words that add depth and provide meaning to the textual content. Furthermore, it trains algorithms to identify the tagged items and improves search queries.
Sentiment Annotation
The field of emotional intelligence in machine learning is hard to implement. Finding actual emotion behind the text is not easy for humans, so expecting machines to do the job requires great expertise and rigorous training. Sentiment annotation or analysis is a process of tagging attitudes, opinions, and expressions within the text.
Machine learning models are trained using sentiment-annotated text data that human annotators label after analyzing and evaluating the text deeply. For instance, analyzing customer reviews requires annotators to read and label reviews into positive, neutral, or negative categories. An accurately trained model can detect user sentiments from social media posts, emails, reviews, comments, etc.
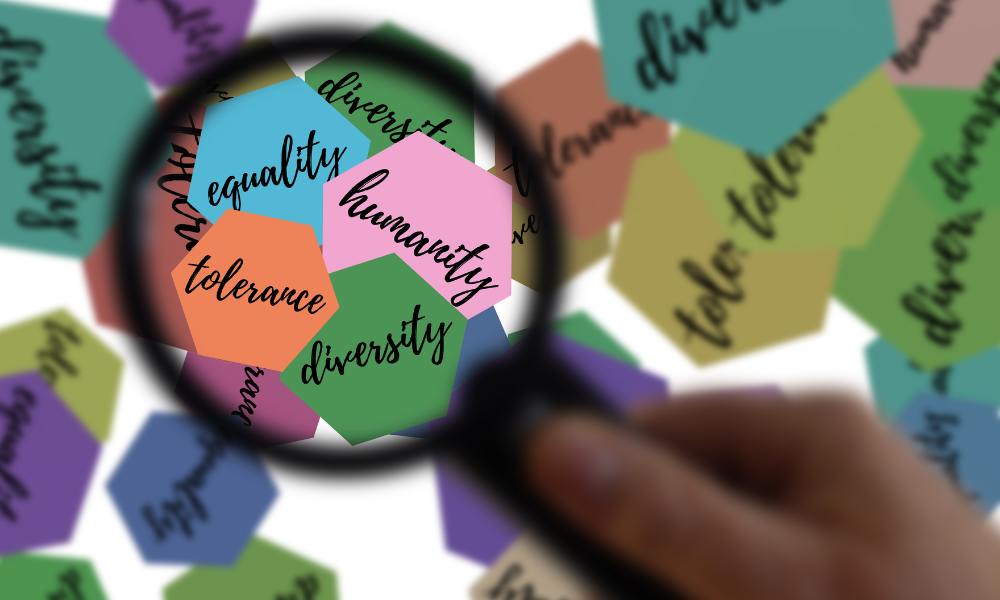
Significance of Sentiment Analysis
In the technologically-driven world, humans are empowered individuals as service users or consumers. They express their thoughts, feelings, opinions, and ideas openly and freely, practicing consumer rights and raising their voices where needed. Therefore, sentiment analysis tools are becoming popular for tracking and translating human sentiments from different data sources.
Boost Business Productivity and Sales
Sentiment analysis helps analyze customer responses, surveys, and feedback to determine why they are happy or unhappy at any point. The sentiment analysis model facilitates businesses to monitor public opinion about their products in client feedback, enabling the company to analyze the trends and demands, plan effective methodology, design and develop future strategies or change current strategies. This process helps companies gain insights to drive useful business decisions and boost revenue.
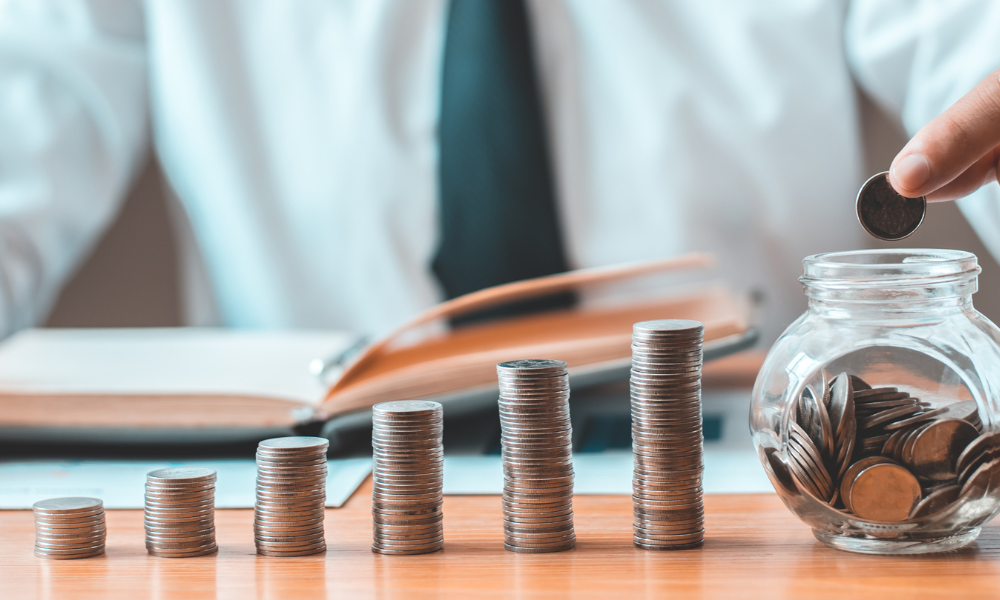
Sort Large Amount of Data
With millions and thousands of tweets, client support conversations, and comments posted daily, manually sorting huge business data is impossible. Most of the data produced by companies are initially unstructured, which needs processing. Sentiment analysis lets organizations process and refines tons of unstructured data efficiently and cost-effectively.
Perform Real-Time Analysis
Sentiment analysis allows businesses to detect serious issues in real-time. For instance, resolving an escalating issue on social media or assessing the churn of dissatisfied customers to devise the right plan and take action immediately.
Social Noise and Its Impact on Lives
The trending social media applications and rising use of technology have encouraged social interactions and expressions online. Observing the user inclination, big brands and companies have also turned towards social platforms like Facebook, Instagram, Twitter, etc., to create their digital business presence.
The growth of online social conversations has led to the emergence of the social noise phenomenon. Sometimes factors like personal or societal influence can confuse or distort the real intention behind the message, creating social noise. People often change their responses or actual opinions influenced by peers or to be a part of the acceptable social network group.
According to the latest stats, sharing and liking misinformation or fake news is becoming a common concern. Users often tend to like or share information or reviews posted by friends and family as a supportive gesture, even if they don’t agree with the idea. Similarly, users avoid liking or supporting opinions that conform to their beliefs just because their social network might disapprove.
Building strong connections, maintaining relationships, seeking people’s approval, creating a personal reputation, affirming your identity while building confidence, and avoiding conflicts are some of the reasons behind the user’s response. Therefore, observing social media information doesn’t guarantee actual reflection of users’ beliefs and opinions, leading to social noise.
One way to combat social noise is through text annotation, which allows us to track and find patterns in the text. This process involves marking up text with AI techniques. By using these methods, we can more easily identify important information and filter out the noise.

The use of AI text annotation is growing in popularity as a way for companies to monitor social media and get a better understanding of the tone around their brand. By being able to see not only what is being said, but how it is being said, companies can more easily identify and address any negative sentiment that may be brewing.
This technology can also help with customer service by providing feedback on how customers are feeling about interactions with company representatives. Are you using AI text annotation to monitor social media chatter? What has been your experience so far?
About the Author:
Aziz Khan is a Solutions Architect at Summa Linguae Technology specializing in delivering profitable solutions for clients.
When Aziz is not working, he spends time in the gym, researching technology, and working on his blog.