Foundation Models, Lock In, and LLM portability

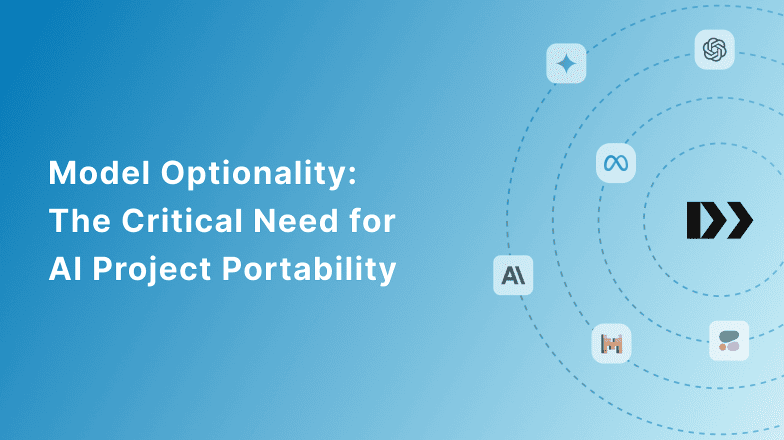
Model Optionality: The Critical Need for AI Project Portability
2024 has been a wild ride for AI. While OpenAI has long been a dominant force, a flood of other open-source and proprietary models have caught up, challenging the status quo. Gemini, Claude, Llama, and Mistral are just a few examples of models that have caught up to OpenAI's capabilities.
As the AI ecosystem continues to mature, it's becoming increasingly clear that model specialization is the future. This trend, while promising, also introduces new challenges for organizations. As models become more specialized, the risk of vendor lock-in increases. To mitigate this risk, it's imperative to prioritize AI project portability.
Why Portability Matters
- Adaptability to an Evolving Landscape: The AI landscape is dynamic. New, more powerful models are constantly being released. Portability ensures that your AI projects can easily adapt to these advancements, maximizing their potential.
- Reduced Vendor Lock-In: By avoiding reliance on a single vendor, organizations can minimize costs and maintain flexibility.
- Optimized AI Efficiency: A portable AI infrastructure allows for seamless model swapping, enabling you to adopt the best solutions as they emerge.
Building a Portable AI Infrastructure
To achieve model optionality, organizations should focus on the following key principles:
- Model Agnostic Design: Develop AI applications that are not tied to a specific model using flexible, API-driven architectures. This allows for easy integration of different models without significant code modifications, making it easier to swap models.
- Standardized Data Formats: Utilize standardized data formats to ensure compatibility across various models and platforms.
- Flexible Training Pipelines: Create training pipelines that can be easily adapted to different models, accelerating the development and deployment process. Exploring ways to make sure your training is portable is critical.
The Future of AI: A Model-Agnostic Approach
As we move forward, a model-agnostic approach will become increasingly important. Organizations should focus on leveraging a robust AI solution that can accommodate a variety of models and minimize the switching costs for maximum optionality. By prioritizing portability and flexibility, businesses can future-proof their AI initiatives and stay ahead of the curve.