IndustryPREP: Harnessing Generative AI for Superior Exam and Interview Preparation
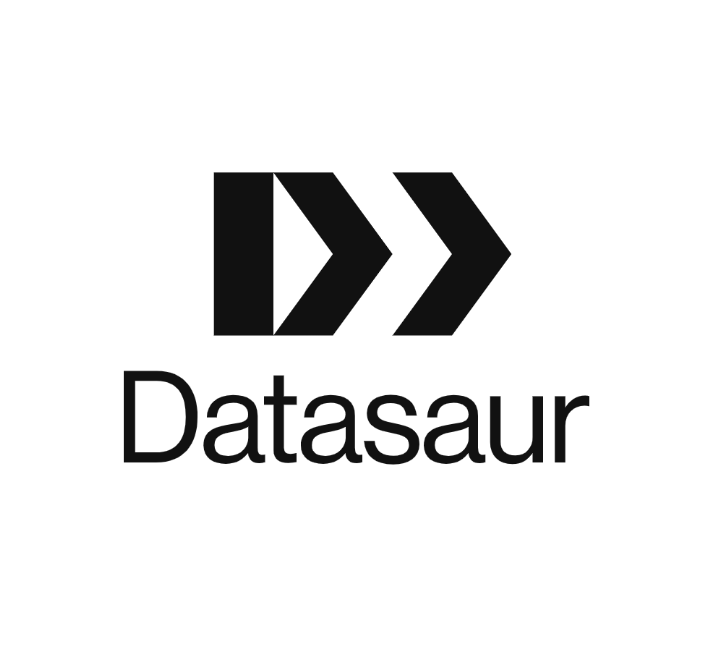
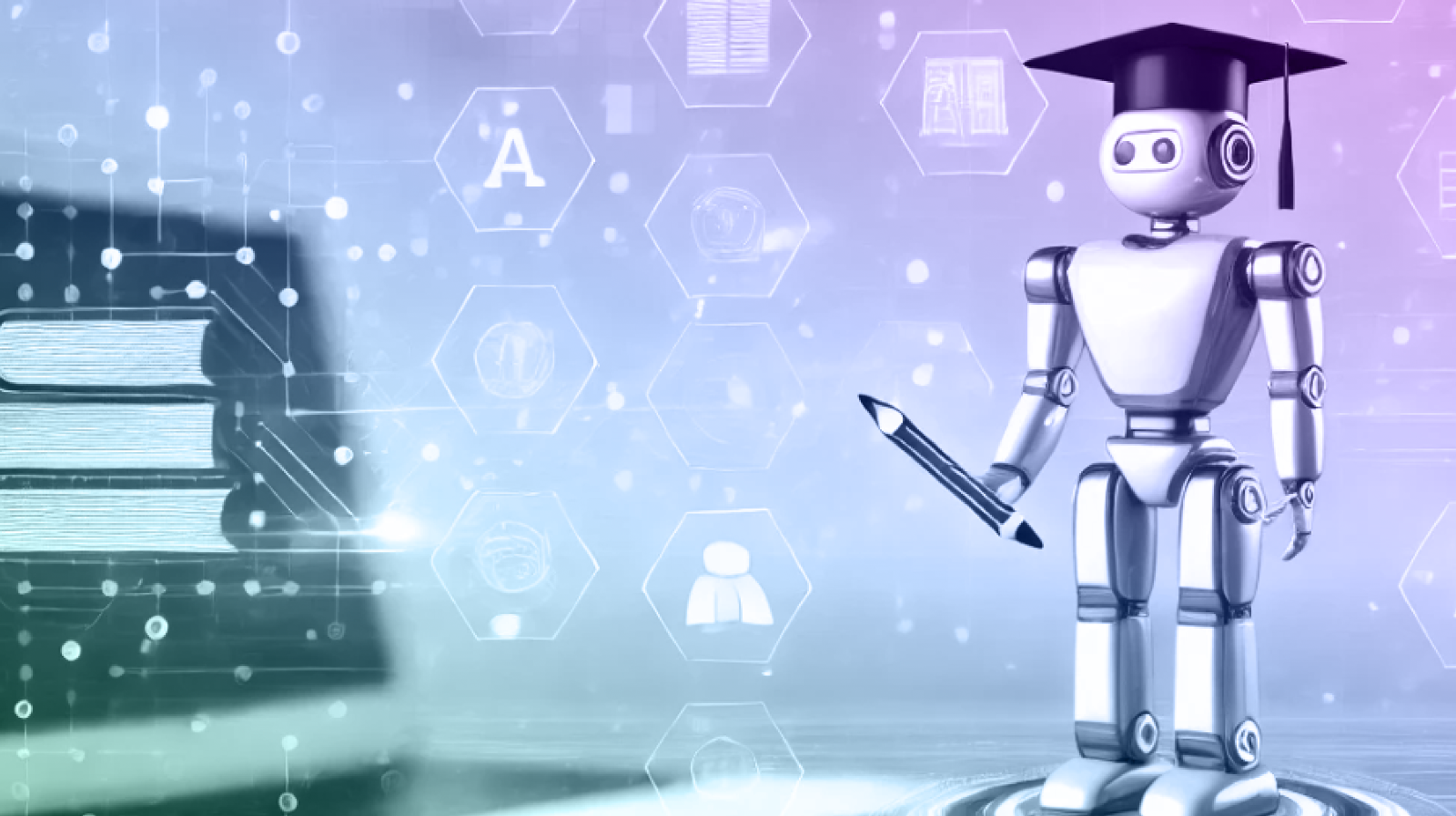
Problem Statement
IndustryPREP is an innovative online platform dedicated to helping students and professionals excel in exams and interviews. Recognizing the transformative potential of large language models (LLMs), IndustryPREP has begun using these models to generate high-quality practice exam questions. While early results have been promising, the company is determined to identify the most effective LLM before fully integrating it into their solution.
Proposed Solution and Architecture
IndustryPREP has primarily used OpenAI's GPT-4 Turbo for most of their generative tasks. To explore alternatives, they experimented with other models like Mistral and Llama. Currently, they leverage LLM Labs to compare multiple models simultaneously, optimizing for speed, answer quality, and cost. This comparative approach allows IndustryPREP to make data-driven decisions on the best LLM for their needs.
Their architecture integrates LLM Labs with a vector store database, containing textbook content hosted on AWS’s S3 bucket. This setup facilitates efficient retrieval and fine-tuning of models, ensuring the generated questions are both relevant and challenging.
Outcomes of the Project and Success Metrics
The primary objective of IndustryPREP's project is to finalize and converge on a foundational model that optimizes speed, quality, and cost. Achieving this objective will enable them to build a robust solution for generating practice exam questions. Key success metrics include:
- Model Performance: Speed and accuracy of generated questions.
- Cost Efficiency: Reducing expenses while maintaining quality.
- Scalability: Ability to connect to a scalable vector store and fine-tune models effectively.
Total Cost of Ownership (TCO) Analysis
Currently, IndustryPREP spends approximately $5000 per month on GPT-4 Turbo. They hypothesize that transitioning to a more cost-effective model could yield similar results at just one-tenth of the current cost. Proving this hypothesis would not only significantly reduce expenses but also enable IndustryPREP to scale their solution across multiple workflows within the organization, driving substantial efficiencies.
Lessons Learned
IndustryPREP's journey has highlighted the vast potential of generative AI in the EdTech sector. Key lessons include:
- Generative AI Potential: Both generating questions and evaluating student answers can benefit immensely from generative AI.
- Importance of RAG: Retrieval-Augmented Generation (RAG) is crucial for tailoring models' knowledge bases to specific educational content.
- Cost-Effectiveness: Optimizing for cost without compromising quality is achievable and beneficial.
Conclusion
IndustryPREP's exploration of LLMs represents a significant advancement in applying generative AI for educational purposes. By optimizing their model selection process and leveraging scalable vector stores, they are poised to deliver a highly effective and cost-efficient solution for exam and interview preparation. This case study not only showcases the potential of AI in EdTech but also provides valuable insights for other organizations seeking to harness the power of generative AI through LLM Labs.