Getting to know Automated Data Labeling

Data labeling simply means sleuthing and tagging information with labels, within a variety of pictures, videos, audio and text assets. Data labeling usually uses human power to create tags and sometimes tags are created with the assistance of a computer. Data labeling for machine learning (ML) teaches Artificial Intelligence (AI) to learn from the labeled data and eventually implement the knowledge it has gathered in real-time scenarios.
Why is Data Labeling Important?
For any AI to work smoothly, the one thing it needs is a mammoth of data. Studies showcase that 80% of the effort in creating an AI is the procurement of useful data. For instance, data labeling is important for visual perception AI models (like self-driving cars, drones or robots) that need annotated images in order to understand the environment and take actions accordingly.
A major role of data labeling is around making objects distinguishable to machines via Computer Vision. Image annotation is the approach used to annotate the images using tools and software. This is a process that requires a huge amount of time and effort to produce the machine learning training datasets required.
When it comes to data labeling, there are two approaches: automated data labeling and manual data labeling. Both have their strengths and weaknesses and which one you choose will depend on your specific needs. Even though manual data labeling is still heavily relied on, technology is moving towards a completely automated system.
What is Manual Data Labeling?
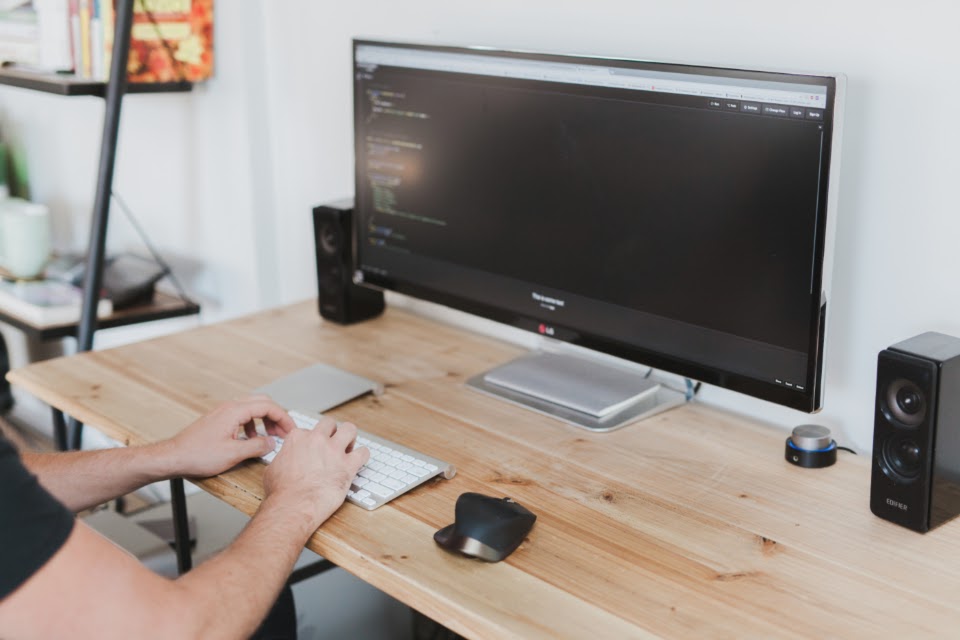
The manual data labeling seems fairly simple but it is monumentally time-consuming. It requires immense skills and precision to manually annotate the objects in the images. Annotators are presented with a series of raw, unlabeled data like text, images, or videos and are given the responsibility of labeling it according to specific data labeling techniques.
Bounding box or polygon annotations are the most commonly used techniques, these techniques are one of the simplest and least consuming techniques in data labeling. Semantic segmentation tends to consume more time compared to fine-grade annotations. In the manual data annotation process, objects are chosen from a specific set of data.
If compared to the automated labeling process, one would assume that it takes a user 10 seconds to draw a bounding box around an object and select the object class from a given list. For instance, if the datasets of 100,000 images with 5 objects per image are available, it would take approximately 1,500 man-hours to label, costing around $10K to label data. Since all of the labeling is done manually, there is a huge opportunity for human error.
How Does Automated Data Labeling Work?

As discussed above, manual data labeling consumes a lot of time and effort is overall a tedious process. To improve efficiency, one must understand which processes can be automated.
Data labeling experts create AI that labels raw, unlabeled data. A human identifies and verifies the label. If the AI (Auto-label) model is successful in labeling the data correctly, it is added to the pool of labeled training data. In case data labeled is incorrect, the information collected from the AI is considered valuable for re-training the Auto-label AI. This is where a human labeler steps in, they will proceed to correct the errors on a trial basis.
Once the errors are corrected and the data is labeled properly, this data is further used to re-train the Auto-Label AI and is eventually tallied to the pool of labeled training data. The final step is taken by the ML teams to use the compiled labeled training data to further train the various models.
Even after this process, the Auto-Label AI needs supervision. Data labeling is at the level where the entire process can be called automated, but humans need to run interference to ensure that the AI does not pick up bad habits. Even AI models like autonomous vehicles need a colossal amount of training to reach the level where it can actually be used safely. Car accident information and labeled data is needed to continually improve model performance.
Data labeling is an integral part of the AI process. AI is always as good as the data fed to it. Data labeling turns raw information into data that makes sense. AI further consumes this data and looks for patterns. This is why there are several training models created for the AI to absorb information to handle scenarios. There is still a lot of manual labor required in data labeling. This is where our data labeling experts come into play. As more accurate data is fed to the auto-label, the more precise it can get.
We have reached a point where a lot of the data labeling process can automated. There is still much left to gain before the entire process is automated, though. Once we reach the point where we can completely automate data labeling, AI will evolve into a new being. General AI will become more of a goal than a fantasy.
Want to learn more? Read this article about why data labeling is significant.