Choosing the Right AI for Legal: Datasaur's Ultimate Benchmark Guide


Choosing the right AI model for legal tasks means navigating a complex trade-off between accuracy, cost, and speed: Datasaur cuts through the noise with a legal benchmark that delivers clear answers. To simplify this, we developed a specialized legal dataset benchmark that provides clarity for legal professionals when evaluating AI solutions.
Datasaur’s benchmark combines real-world court-derived questions with the LegalBench evaluation suite to measure LLM performance in legal contexts. LegalBench is a widely used tool for testing how well models perform on domain-specific reasoning tasks. By integrating these two sources, we ensure our benchmark reflects both theoretical robustness and real-world applicability. This combination allows us to evaluate not only accuracy, but also reasoning and retrieval depth—key factors for legal professionals.
To simulate actual legal workflows, our benchmark includes complex, jurisdiction-specific questions drawn directly from court filings and opinions. For example:
Explain the legal standard applied by the court when considering a motion to dismiss for "failure to state a claim upon which relief can be granted," and identify the key cases cited in the document that support this standard.
What opportunity has the court provided to the Plaintiff regarding the business expense reimbursement claim, and what specific information is the Plaintiff required to include in the amended complaint?
These questions require the model to both retrieve information and apply multi-step reasoning. This ensures we test beyond surface-level summarization or keyword matching.
Model Comparison: Balancing Accuracy, Cost, and Speed
We evaluated five leading language models—GPT-4o, Claude 3.7 Sonnet, Gemini 1.5 Pro, DeepSeek-V3, and Llama 3.3 70B—using DeepSeek R1 as our impartial evaluator. DeepSeek R1 acted as an LLM-as-a-judge, a common strategy in recent AI research to generate consistent and scalable scoring
Claude 3.7 Sonnet stood out by achieving the highest correctness score, with the score of 8.62 against the benchmark questions. However, this precision comes with the highest cost, approximately 0.6 USD to run on our benchmark dataset. On the other hand, DeepSeek-V3 emerged as the most cost-effective model at 0.05 USD to run on our benchmark dataset — Which almost 10 times cheaper than Claude 3.7 Sonnet, yet it had a lower correctness score of 8.41 — roughly 0.2 point below the Claude 3.7 Sonnet.
This trade-off between accuracy, cost, and speed emphasizes the need to match model selection with your use-case priorities. High-stakes legal workflows—such as due diligence or litigation research—may require the precision of Claude 3.7 Sonnet. Meanwhile, scalable use cases—like summarizing hundreds of routine filings—may benefit more from DeepSeek-V3 ’s affordability. Defining these priorities upfront ensures you choose a model that aligns with your operational goals.
Claude 3.7 Sonnet stood out by achieving the highest correctness score… On the other hand, DeepSeek-V3 emerged as the most cost-effective mode
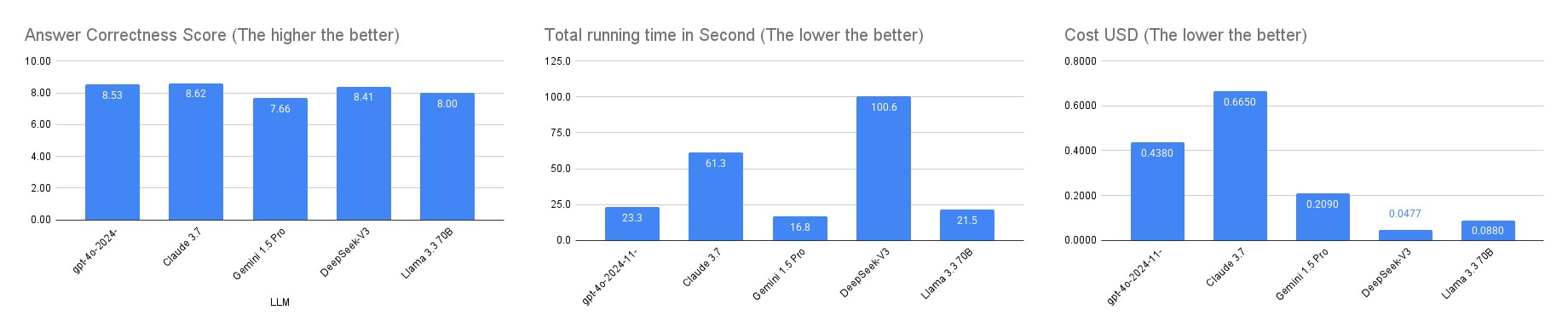
Precision Matters: Custom vs. Automatic Evaluation
At Datasaur LLM Labs, we utilize both Automatic and Custom Evaluations to ensure our benchmarks are accurate and reflective of real-world applications. While Automatic Evaluation provides a quick, initial assessment of model performance, we found discrepancies between automatic scores and practical usability.
For instance, an Automatic Evaluation might score a response at 5.0, while a more nuanced Custom Evaluation—with manually refined prompts—could higher that score to 7.0. This custom approach allows us to incorporate domain-specific nuances, aligning the model evaluations closer to actual expert judgments.
Example of a custom evaluation prompt we used:
Role:
You are an AI legal evaluator assessing the accuracy of a response based on the provided prompt, expected completion, and completion.
Task Description:
When one company sells its assets to another company, the purchaser is generally not liable for the seller’s debts and liabilities. Successor liability is a common law exception to this general rule. In order to spot a successor liability issue, lawyers must understand how courts apply the doctrine.
The doctrine holds purchasers of all, or substantially all, of a seller’s assets liable for the debts and liabilities of the seller if:
1. the purchaser expressly agrees to be held liable;
2. the assets are fraudulently conveyed to the purchaser in order to avoid liability;
3. there is a de facto merger between the purchaser and seller; or
4. the purchaser is a mere continuation of the seller.
Express agreement is governed by standard contract law rules. In practice, if a purchase agreement contains a provision to assume liabilities, litigation will rarely arise. Courts, however, sometimes interpret express agreement in the absence of a written provision.
Assets are fraudulently conveyed when the seller intends to escape liability through an asset sale or knows that liability will be avoided through an asset sale.
De facto merger is a multifactor test that consists of (1) continuity of ownership; (2) cessation of ordinary business and dissolution of the acquired corporation as soon as possible; (3) assumption by the purchaser of the liabilities ordinarily necessary for the uninterrupted continuation of the business of the acquired corporation; and (4) continuity of management, personnel, physical location, assets, and general business operation. Some jurisdictions require a showing of all four elements. Others do not and simply emphasize that the substance of the asset sale is one of a merger, regardless of its form.
Mere continuation requires a showing that after the asset sale, only one corporation remains and there is an overlap of stock, stockholders, and directors between the two corporations. There are two variations of the mere continuation exception. The first is the “continuity of enterprise” exception. In order to find continuity of enterprise, and thus liability for the purchaser of assets, courts engage in a multifactor analysis. Factors include: (1) retention of the same employees; (2) retention of the same supervisory personnel; (3) retention of the same production facilities in the same physical location; (4) production of the same product; (5) retention of the same name; (6) continuity of assets; (7) continuity of general business operations; and (8) whether the successor holds itself out as the continuation of the previous enterprise. The second is the product line exception. This exception imposes liability on asset purchasers who continue manufacturing products of a seller’s product line. This exception generally requires that defendants show that the purchaser of assets is able to assume the risk-spreading role of the original manufacturer, and that imposing liability is fair because the purchaser enjoys the continued goodwill of the original manufacturer.
Scoring Criteria:
- Evaluate the response based on the task description.
- Assign a heavier weight to whether the response correctly identifies an exception.
- Consider the reasoning provided as a secondary factor that influences the final score.
- Score the response on a scale of 1 to 10 (integer values only).
Output Format:
- The score should be enclosed in double square brackets.
- Example output: [[9]]
This tailored method notably enhances the precision and applicability of the evaluations.
Concluding Thoughts
Claude 3.7 Sonnet is best suited for legal tasks that demand high-precision and minimal tolerance for factual errors. This includes legal research, regulatory analysis, and opinion drafting. For budget-conscious workflows—such as triaging or summarizing standard documents—DeepSeek-V3 offers exceptional value. By understanding this trade-off, legal teams can deploy the right model for the right task, optimizing both performance and ROI.
Take the Next Step
Explore Datasaur LLM Labs and gain more profound insights into our benchmarks. Choose the best AI model tailored precisely to your legal workflows. Find the best-fit model for your legal workflows—accurately, quickly, and cost-effectively with Datasaur.