9 Reasons Datasaur.AI is the Best Text Annotation Tool

AI and ML models rely heavily on reliable sources of data for learning patterns. Data labeling is critical to help the models understand the data they are fed. In this article, we will take a look at text labeling and how AI uses it to tell you stories, and generate blog posts or help you write an email to your potential client.
Data annotation or labeling tools are expected to cross over $25 billion by 2032. Datasaur.ai has taken a step forward by creating a digital data labeling toolbox. Datasaur.ai brings one of the most customizable platforms for NLP labeling at a remarkably fast pace.
To help you get the most flexible data annotation tool, we discuss Datasaur’s robust, collaborative text annotation, and labeling paradigm, and how it beats the competitors in the field.
Why Is Datasaur.AI the Best Text Annotation Tool?
Datasaur.ai has built a data labeling platform optimized for Natural Language Processing (NLP) to help computers and digital systems understand, interpret, and handle natural human language. Something that other annotation tools lack. Project-specific labeling schemes are a breeze with Datasaur.
Datasaur.ai’s advanced NLP tool for data annotation is designed to manage the most complicated requirements. It can enhance model performance by double and drive 10 times quicker project times.
1. Quality Control Management
Quality control is essential for text annotation tools. Top-quality data results in the development of an equality model where issue identification of issues is possible at the root level.
Datasaur cares about the text annotation quality. Datasaur.ai's QA capabilities provide detailed and high-level reviews of labels and labelers guaranteeing data quality. It controls the complete quality management stream with accelerated outputs at 10X improved project deliveries.
2. Integrated annotation comparison
Integrated annotation comparison is the method that is used for comparing different annotations of the same dataset drawn by many different annotators employing various labeling techniques. Moreover, it allows for identifying differences and evaluating the quality of overall labeling.
The annotation comparison process is important for ensuring the validity, accuracy, and reliability of the labeled dataset, which results in enhanced performance of machine learning models.
3. Process Automation/ Redundancy Reduction
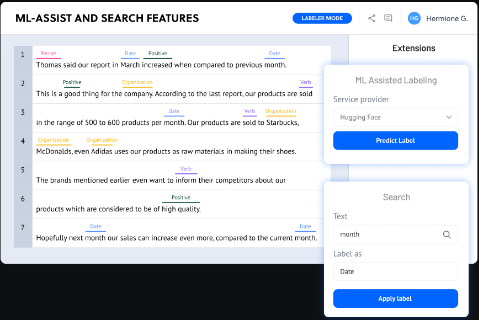
Typically, labeling text data is a manual task, which may involve performing the same set of steps repeatedly. It can lower productivity while increasing time consumption. Therefore, Datasaur suggests reducing repeatable cleaning and manually labeling documents by automating highly repetitive tasks.
When you automate routine tasks, it leads to boosted performance. Moreover, saves time and effort for your team workers while allowing them to concentrate and work on building enhanced models in that duration. Datasaur automates text annotation workflows in bulk, covering entirely from project configuration and export to labeling.
4. Text Annotation Customization
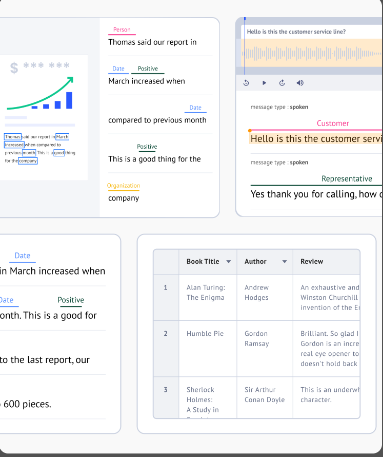
When teams work on customizing heavy text annotation tools or trying to expand feature requests for satisfying user requirements, it can massively drain your resources. Relying on customizable workflows and a genuinely configurable web user interface can help resolve the issues. In case you need to switch tools, use file transformers or converters for ease in transfer, configuration, and export from Datasaur.
Datasaur helps automate project creation and export, and control access levels to maintain a smooth flow. Our human customer support team is always on standby to receive and understand customized feature requests. With the Datasaur team on your side, all your customizations will be served in the annotation export format you want.
5. Error Elimination
Errors are unavoidable in collaborative labeling and it's not easy to trace them. You often have to spend hours figuring out the errors manually by going through the annotation files. If you have no clue about the bug or knowledge of the techniques, you clearly have a daunting problem on your hands.
Datasaur provides proper and advanced labeling stats to review the performance and gain insights about the structured data. Additionally, Datasaur's QA features help you find details at a granular level.
6. Project Lifecycle Acceleration
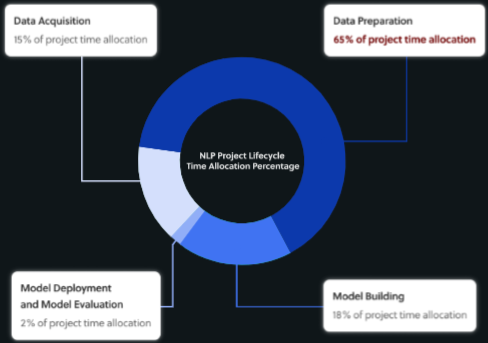
A slow and sloppy annotation process is never desired. Most of the time in a project lifecycle is consumed in the preparation phase. It involves the cleaning and annotation task which can be exhausting for individuals.
Datasaur understands the pain and brings an effective method to accelerate the entire NLP project lifecycle by intelligently automating 80% of your process. It fastens up the development phase without affecting the validity and quality of your ML models. On top of this, it offers advanced tools for the entire NLP data labeling workflow covering ML-assisted labeling services to top-quality QA capabilities.
7. Comprehensive Audio Labelling
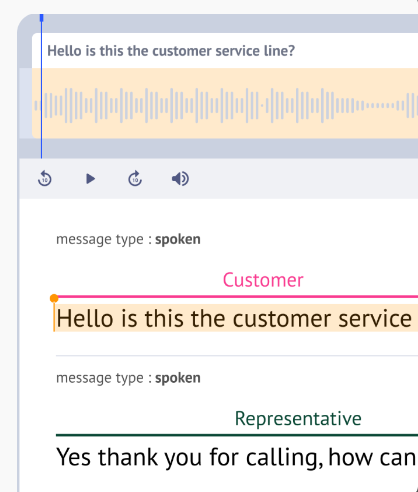
Users often have to deal with audio in the form of large files or phone call conversations. Annotating such files is not a simple task. Datasaur brings user-friendly tools to help transcribe audio, conversations, and calls while labeling. It allows editing transcriptions, using timestamps, providing multi-language support, and other options for improving the workflow. You will be able to isolate where a label occurred both in the transcription and actual audio.
8. Advanced Workforce Management
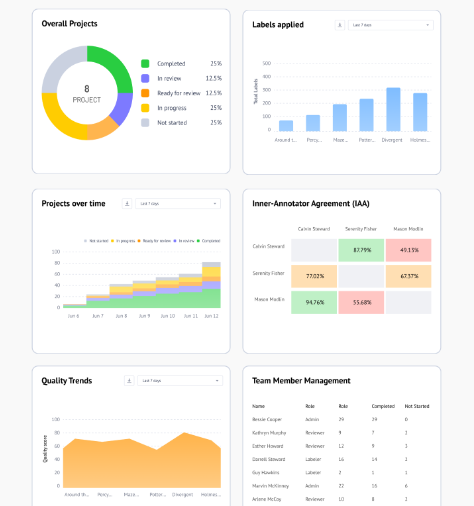
7. Workforce management is the key to analyzing and boosting performance. Datasaur offers dashboards for a high-level project view to see individual labeler progress and remove any barriers. It lets you pull reports, run QA, and identify disagreements between annotators to rule out problems easily.
Datasaur further allows you to visualize analytics at the levels of team, project, and individual. It provides a clear picture of the internal procedures to know what’s happening at every level for your projects. You can create QA reports, check detailed dashboards, and resolve issues between annotators in a few clicks.
It helps you detect obstacles just as they arise and easily access any particular insight for keeping the timelines on record. You get all the management tools covering your access management, project allocation, and task division all under one platform.

Advanced NLP Labeling tools and stats are quite helpful and handy for easily managing your most complex labeling requirements. Datasaur offers textual classification, entity extraction, entity linking, multiple-layer labeling, bounding box labeling, and OCR all while using the language of your choice: left to right or vice versa.
Datasaur supports quick and efficient labeling with a robust pack of NLP tools. You get convenient-to-use labeling features that make labeling feel less like a laborious deal. You can use Datasaur’s ML-assisted labeling tool which enables you to plugin models from Huggin Face, OpenAI, your own model, and more. You can also upload pre-labeled datasets, bulk label, and highlight inconsistencies or typing errors within the data.
What sets Datasaur.ai apart from its competitors?
Datasaur focuses all its attention on natural language processing, text annotation, particularly and works on refining it over time. While other annotation tools are trying to be the jack of all trades and master of none in the A space. Free text annotation tools don't even come close to our capabilities.
Moreover, it offers robust tools for entity linking, multiple layers of labeling on a single token, sentiment analysis, intent labeling, PII anonymization, OCR, etc. The best thing is that it lets you label and transcribe input documents and text files in any language of your choice.
Some of its outstanding features include:
Military-grade Security
- VPC and on-premise deployment options
- E2E encryption
- SOC2 / HIPAA certified
Seamless Integrations
- Automatic project creation and export
- AWS, GCP, and local storage
- Modern User management platforms (SAML, Google SSO, etc.)
Hassle-free Deployments
- Datasaur-hosted on AWS
- Public cloud of user choice
- On-premise or VPC deployment
It offers timeless applications in the legal, finance, healthcare, and eCommerce departments.
Frequently Asked Questions
What Is Text Labeling?
Text annotation refers to the identification of raw data in the form of various text files. Informative tags and labels allow machine learning models to learn patterns and attributes about the data.
It is an essential stage in preprocessing where labeled data is required for classification as both input and output by data scientists. You can either perform manual annotation or use any reliable software.
Text labeling involves text classification where labels are assigned to text files. It highlights words and adds metadata against important language content. The labeling scheme may differ according to the software features and user needs. The labels assigned should be informative and differentiating.
What Are Important Factors for Data Labeling?
Different factors impact the labeling process. Therefore, no single method can be considered optimal for labeling data. Enterprises must select the technique or combination of techniques that best suits their requirements.
While picking a data labeling scheme or technique, consider a few important factors given below:
- the goal of the ML model demands labeled data
- the size of the firm
- the size of the dataset that requires labeling
- the skillset level of employees
- the financial restraints of the firm
The text annotation team must have domain knowledge and know-how of the industry. Additionally, data labelers must be flexible and quick, considering the iterative and agile nature of data labeling and ML processes which are constantly changing and evolving with the information.
What Are the Uses of the Datasaur Text Annotation Tool?
Here is a list of some use cases for our scriptable annotation tool.
- Named Entity Recognition
- Text Classification
- Sentiment Analysis
- OCR
- Active learning
- Document Labeling
- Data Extraction
- Speaker Categorization/Diarization
- Entity Disambiguation
- Entity Linking
- Part of Speech
- Coreference Resolution
- Audio Labeling
Conclusion
Datasaur.ai is an annotation tool that specializes in text annotation and is dedicated to bringing the best user interface and experience in the industry. It is an incredible remedy to manual annotation and a dream come true for data scientists to share their vision with the team via Datasaur's basic diagrammatic overview.
Using an easy-to-use annotation tool and a properly labeled dataset delivers a baseline to evaluate the ML model predictions for accuracy and continuous refinement of the algorithm. It’s time to stop draining your energy while trying hard to make any ordinary annotation tool fit your requirements forcefully. Instead, build scalable data labeling flows that are simple, effective, and truly fit what your team needs.
Author Bio:
Aziz Khan is a Solutions Architect at Summa Linguae Technology specializing in delivering profitable solutions for clients.
When Aziz is not working, he spends time in the gym, researching technology, and working on his blog.