Datasaur - Now Supporting LLMs
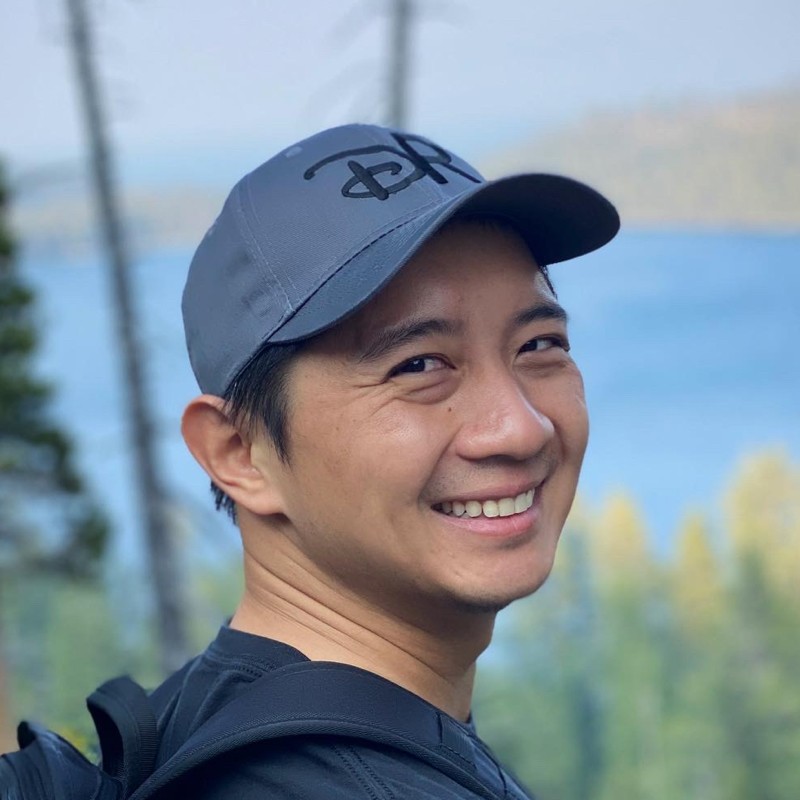
When I started Datasaur 4 years ago, I had personal experience building dozens of NLP models at a number of different companies. The single most frustrating portion of that process was creating the right training dataset. I founded this company to streamline the labeling process and help teams around the world adopt best practices in their labeling.
This year, NLP has taken center stage with an emphasis on ChatGPT and LLMs. Professionals across every industry are wondering how to best leverage this technology for their own work. Once again, there’s a lack of clarity and industry standards on how to best build and train your own custom models. People we talk to are confused about how to finetune and improve one of the many, many open source models. The industry is ever evolving and changing, but we wanted to quickly launch what we do best - support you in gathering your training data.
In Datasaur’s LLM Evaluation tool, you’ll have the opportunity to assess your model’s current status - do the answers meet the expectations for your business use case? You can rate answers from 1 to 5 - anything less than a 5 will ask you to provide your expected completion. This can be used to help assess the model’s performance (we recommend scoring at least 85% across all prompts) while also providing answers that can be fed back into the model for further improvement.
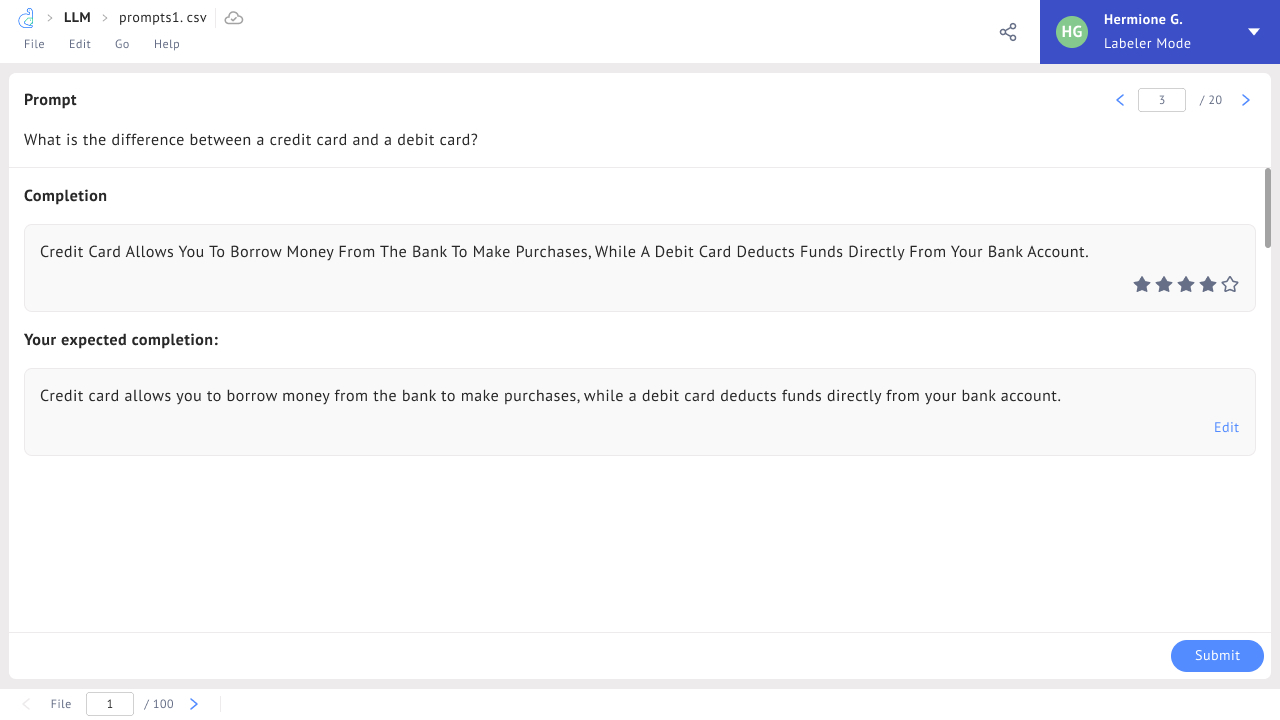
In Datasaur’s LLM Ranking tool, you’ll find everything you need to help with your own Reinforcement Learning from Human Feedback (RLHF) process. A prompt will be displayed alongside 3 completions from the LLM, and you’ll need to rank them in order. The results of this ranking process can be used to train a reward model that is crucial to the RLHF process (we recommend the open-source library trlX).
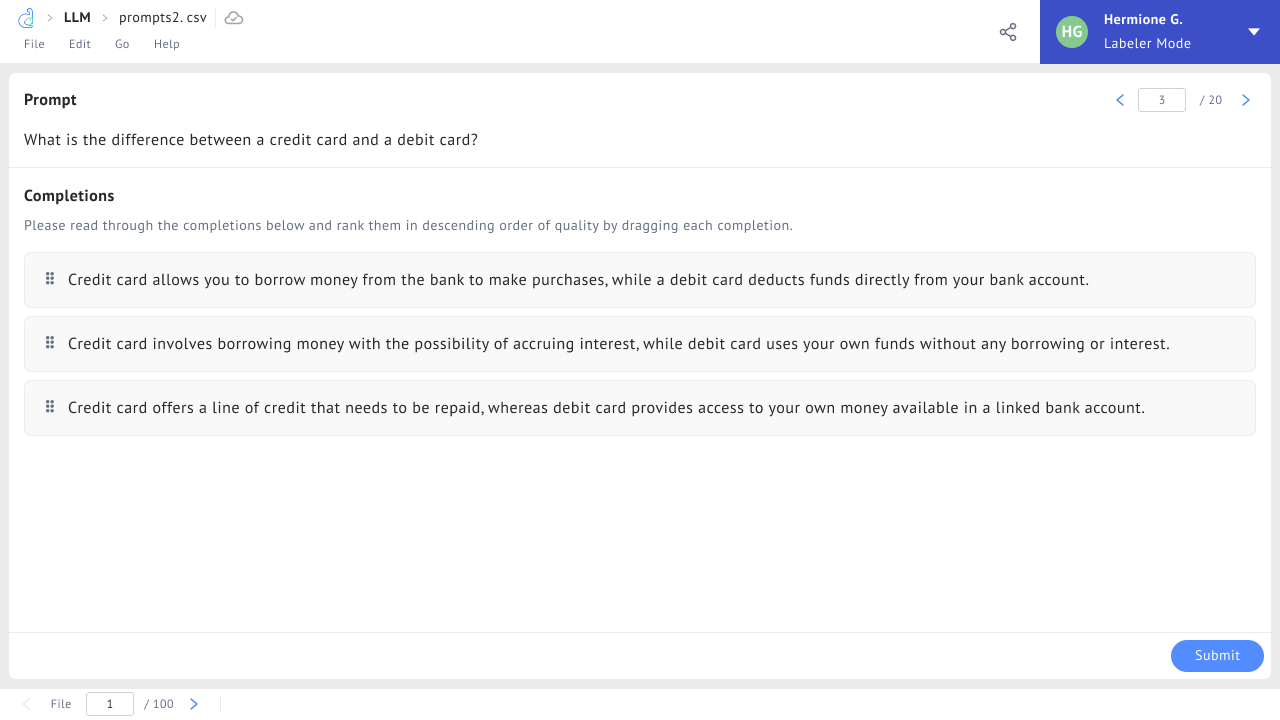
We spent hundreds of hours interviewing and working with a number of cutting edge researchers and developers to produce a project interface that is both incredibly intuitive and easy for an annotator or subject matter expert to learn, while also catering to the data scientist as the end user. The projects can be exported directly to formats that can be used for fine tuning and RLHF consumption.
And of course, all of this leverages Datasaur’s industry-leading Reviewer mode and automatically calculates Inter-Annotator Agreement to ensure you have full insights into the quality and efficiency of your work.
We’re excited for you to give this a whirl. Send us an email at demo@datasaur.ai to try it out!