Empowering Medical Research with Precision Data Labeling
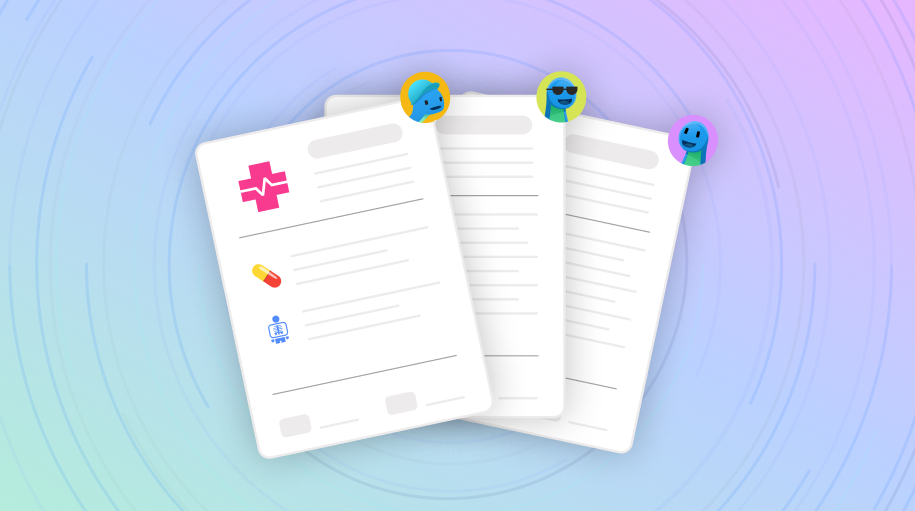
Deciphering and organizing people's radiology reports is a task that requires meticulousness, and is not without complications or issues. Radiology reports consist of free text containing crucial information about a patient’s health based on doctor interpretations of radiology images and patient clinical history. However, the unstructured nature and complexity of language in the reports create challenges when using radiology reports for clinical research, especially in settings with limited labeled data.
To navigate these obstacles in healthcare research, Harvard and Stanford researchers including Andrew Ng, utilized Datasaur’s data labeling platform. In their published research article presented at the 35th Conference on Neural Information Processing Systems (NeurIPS), the article states that traditional methods to extract information from radiology reports have their limits.
While automated systems such as MIMIC-CXR and CheXpert excel at identifying common medical conditions in vast datasets, medical researchers reported that they often miss crucial details embedded within these reports, such as finding specific entities and their relations. The insufficiency of existing approaches in organizing data information prompted researchers to think outside the data labeling box.
Using the Datasaur data labeling platform, researchers were able to extract a broader range of information from the radiology text using a new information extraction schema designed for report coverage and generalizability.
Datasaur’s robust NLP platform provides tooling for many text/language workflows including entity extraction that non-technical users can easily utilize and manage. This is one reason why Datasaur is a great platform for such medical use-cases. Users can create any taxonomy on Datasaur and apply their custom labels of that taxonomy to the dataset. In fact, you can create taxonomies that are unique to identify relationships between labels, as this research team demonstrated in the graphic below. Medical teams can upload large batches of data and label, either through manual or automated means, the tokens within the dataset. This is what enabled Harvard and Stanford to deploy their custom schema to these radiology reports.
Not only can you manually label with your custom taxonomy on Datasaur but you can also introduce automation to your labeling process. Once you have created early versions of your ML Model, we can integrate with your model to automatically apply labels to your unlabeled dataset (as seen in the graphic below). In fact, Datasaur has recently created a new feature, Dinamic, that streamlines this process by enabling users to create a model based on your labeled data directly from the labeling interface. Datasaur is designed for technical and non-technical users alike to access the latest innovations of the NLP and LLM industry: empowering you to label your data efficiently and accurately.

At Datasaur, we're not merely facilitating data labeling—we're empowering users with tools to push the boundaries of informational organization in their pursuit of medical knowledge.
Special thanks to researchers Saahil Jain, Ashwin Agrawal, Adriel Saporta, Steven Truong, Du Nguyen Duong, Tan Bui, Pierre Chambon ,Yuhao Zhang, Matthew Lungren, Andrew Ng, Curtis Langlotz, and Pranav Rajpurkar for using Datasaur labeling services. To read the full report visit here.